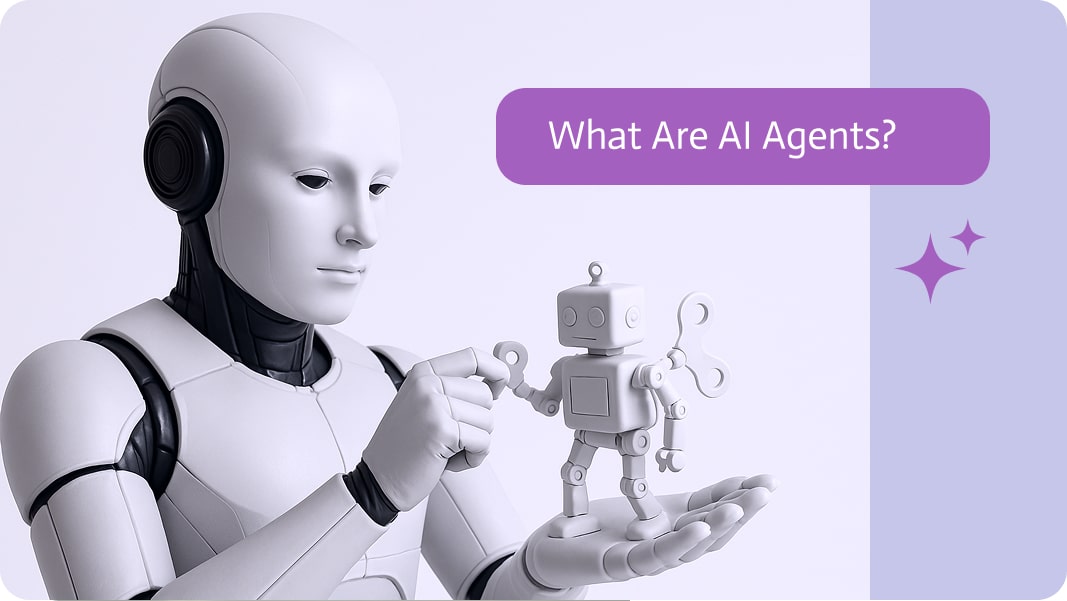
What Are AI Agents?
Unleashing the Power of AI Agents: Driving Innovation and Efficiency
AI agents are intelligent software entities designed to perceive their environment, make autonomous decisions, and take action to achieve specific goals. Operating on principles rooted in artificial intelligence (AI), they employ techniques such as machine learning, deep learning, and natural language processing (NLP). These agents have become pivotal across industries, driving innovation and efficiency in business operations, customer engagement, and complex problem-solving.
At their core, AI agents act as intermediaries between users and systems, executing tasks autonomously or semi-autonomously based on their programming and learning capabilities. They analyze vast amounts of data, derive insights, and perform actions with speed and precision. Unlike traditional software programs that require explicit instructions for each task, these agents learn and adapt over time, improving their performance through feedback and experience. This autonomy allows them to operate in dynamic environments where predefined rules may not suffice.
In platforms like Noca AI, AI agents revolutionize automation and integration processes, empowering businesses to connect disparate systems seamlessly. By leveraging Noca AI’s capabilities, users can create intelligent workflows that adapt to changing environments, streamlining operations with minimal effort.
The Heart of AI Agents: Core Functions and Mechanisms
Understanding what makes AI agents truly intelligent and effective involves exploring their core functions and underlying mechanisms. These attributes set them apart from traditional software and define their capabilities in real-world applications.
1. Autonomy: The Ability to Operate Independently
One of the defining characteristics of AI agents is their ability to function without constant human intervention. Autonomy means these agents can independently analyze situations, make decisions, and execute actions. For example, in a customer support scenario, an AI agent can assess a user’s query, identify the problem, and provide a solution without manual oversight.
This autonomy relies heavily on advanced algorithms and data processing capabilities. By leveraging historical data and contextual information, AI agents can predict outcomes and adjust their strategies to meet specific objectives.
In Noca AI, this autonomy shines in customer-centric automations. For instance, an AI agent within Noca AI can monitor incoming support emails, classify them based on urgency, and trigger follow-up actions like generating tickets in a helpdesk system or notifying relevant teams—all without human intervention.
2. Adaptability: Learning and Evolving Over Time
Adaptability allows AI agents to improve their performance by learning from interactions and data. Through techniques such as reinforcement learning and supervised learning, these agents refine their decision-making processes and become more effective over time.
For instance, an AI agent managing a supply chain might initially rely on basic data inputs, such as inventory levels. Over time, it learns to account for external factors like seasonal demand or logistical delays, optimizing operations more effectively.
3. Decision-Making: Intelligent Problem Solving
AI agents excel in making complex decisions, often in real time. They utilize algorithms, probabilistic reasoning, and machine learning models to weigh multiple factors and determine the best course of action. This capability is particularly valuable in high-stakes environments like financial trading or autonomous driving, where decisions must be both accurate and instantaneous.
4. Action-Oriented: Executing Tasks Efficiently
After making a decision, AI agents act to achieve their goals. These actions could range from sending notifications or executing commands to physically manipulating objects in the case of robotic agents. The ability to bridge the gap between decision and execution makes these agents indispensable in automating repetitive or time-sensitive tasks.
5. Perception: Understanding the Environment
AI agents gather information about their surroundings through sensors, APIs, or other data streams. This perception layer enables them to interpret complex scenarios, such as understanding user emotions in customer interactions or identifying patterns in large datasets. By contextualizing this information, these agents can tailor their actions to specific situations.
Q&A: Diving Deeper into AI Agents
1. How do agents process conflicting information from multiple sources?
Agents rely on algorithms to reconcile conflicting data by weighing the reliability of sources and identifying patterns or outliers. How they prioritize information depends on their programming and training.
2. What makes an AI agent’s decision-making mechanism superior to traditional algorithms?
While traditional algorithms follow predefined rules, AI agents incorporate learning and adaptability, allowing them to optimize decisions in dynamic and unpredictable environments.
3. Can agents collaborate with each other, and if so, how?
Yes, agents can collaborate by exchanging data and coordinating actions through shared platforms or communication protocols. This is common in multi-agent systems like logistics networks.
4. How do agents balance short-term actions with long-term goals?
Through their planning modules, agents evaluate immediate tasks against overarching objectives, using techniques like utility optimization to align actions with long-term strategies.
5. Are there limits to how much an AI agent can learn and adapt?
AI agents are limited by the quality and quantity of data they receive, the algorithms used, and computational resources. Ethical considerations and regulatory constraints can also impose boundaries on their learning capabilities.
The Future with AI Agents
By understanding the mechanisms and components of AI agents, businesses can leverage their capabilities to drive innovation, improve efficiency, and tackle complex challenges. Platforms like Noca AI amplify the potential of agents, providing businesses with tools to automate integrations, manage workflows, and scale operations with unprecedented efficiency.
As these agents continue to evolve, their role in shaping the future of technology will only become more significant. Whether streamlining enterprise processes or redefining customer engagement, agents are poised to remain at the forefront of innovation.