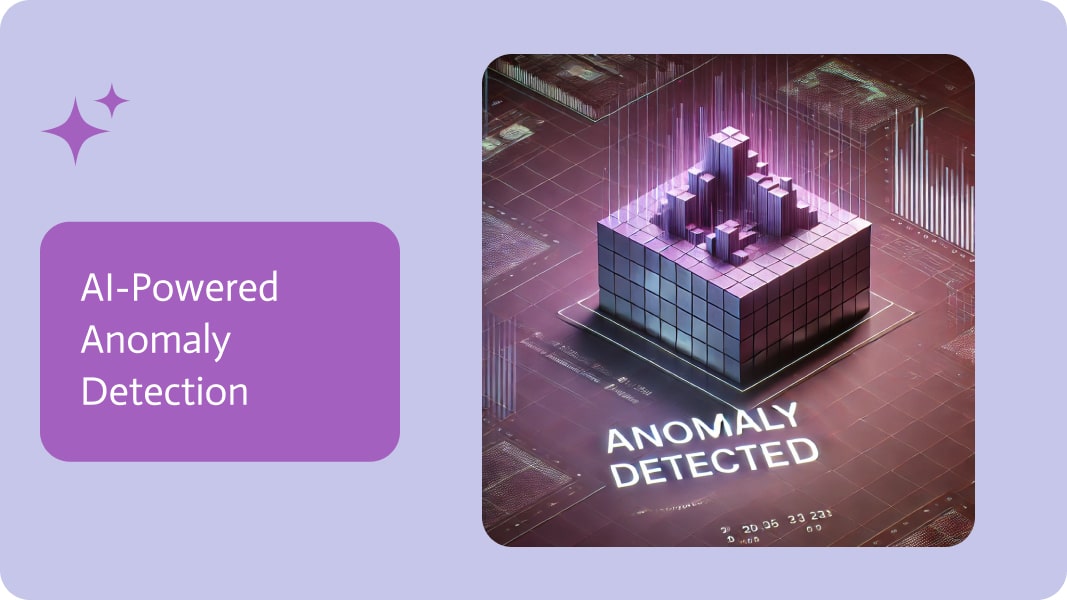
AI-Powered Anomaly Detection
What is Anomaly Detection?
Anomaly detection is the steps of identifying patterns or data that change significantly from the anticipated behavior in a dataset. Deviation is often referred to as ”outliers,” and can signal many different issues. These issues range from operational inefficiencies and system crashes to security breaches. Data is growing in complexity, volume and variety, the limitations of these approaches have become apparent.
Before AI powered anomaly detection the traditional approaches worked well but were only effective in smaller and structured datasets. These traditional detection methods struggle on a larger scale.
How AI Anomaly Detection Actually works
This tool uses high end machine learning techniques to analyze and classify deviations from normal patterns. This process normally involves these steps:
- Data collection- These systems gather data from different sources such as, transactions,Sensors, logs and network traffic.
- Data processing- The data is cleaned, set to normal, and structured to ensure consistency.
- Feature engineering- The main attributes are extracted and transformed to focus on relevant characteristics for anomaly detection.
- Model training- The AI model is trained in historical data which is either though supervised learning or unsupervised.
- Anomaly detection- When the model is trained it will continuously analyze new data in real time, flagging any issues.
- Alert & response- When the anomaly is triggered, automated alerts or actions will be notified, reducing manual intervention.
By using intensive learning architectures like autoencoders, recurrent neural networks (RNNs), and generative adversarial networks ( GANs), AI driven anomaly distinction systems can adapt over time, refining detection and minimizing false announcements.
AI Powered Anomaly Detection Across Industries
AI Powered anomaly detection is changing how industries recognize and respond to unusual patterns or behaviours. When using machine learning algorithms, businesses can spot anomalies more efficient and correctly, leading to improved decision making and risk management.
Healthcare
- detects patient data to recognize unusual health patterns.
- Identifies fraudulent claims and billing anomalies.
- Enhances patient safety by recognizing medical errors.
Fiance
- Recognizes fraudulent transactions.
- Keeps track of trading patterns to detect insider market manipulation or trading.
- Credit risk is assessed by scanning for unusual spending behaviour.
Manufacturing
- Anticipates equipment failures by identifying sensor data.
- Monitors the process of production for deviations from any standard operations.
- Minimizes downtime and potential maintenance costs through early detection of anomalies.
Cybersecurity
- Scans for unusual network traffic patterns of cyber threats.
- Tracks user behaviour to recognize potential internal threats.
- Expands threat intelligence by analyzing historical data for anomalies.
Retail
- Identifies customer conduct to detect fraudulent activities.
- Tracks inventory stock for unusual patterns th ta may be theft.
- Advance supply chain management by spotting anomalies in demand forecasting.
The top 3 AI powered Anomaly Detection tools of 2025
Splunk Enterprise
Splunk Enterprise provides user behaviour analytics which uses AI and machine learning to find hidden threats by scanning behaviour patterns, groups and advanced correlations. It addresses risl ratings to anomalies by helping security teams take correct action.
Features
- Risk based threat scoring with supported evidence.
- AI driven real time detection.
- Multiple industries can use this tool.
- Easy integration with enterprise systems.
Anodot
Anodot is powered by AI and uses anomaly detection to analyze time series data in real time. This tool uses machine learning to identify unusual patterns, correlates data points and manages insights and turns them into actionable decisions. This allows businesses to detect risks early and act efficiently.
Features
- AI driven insights with instant monitoring.
- Detects outliers in data.
- Supports IT and business analytics.
- Removes delays in business commanding.
Cynet
Cybersecurity and AI based platform , Cynet detects and prevents cyber threats. It is always monitoring files, users and network traffic. Cynet then uses AI to spot suspicious activity before it becomes a real issue.
Features
- AI powered threat detection.
- Lowers false positives for improved accuracy.
- Automates security measures to save time.
- Provides real time threat intellect.
Benefits of AI powered Anomaly Detection
Improved Accuracy & Reduced False Positives
AI models continue to learn and adapt, improving their ability to differentiate between actual analysis and variations in data.
Real Time Monitoring & Faster Response
Businesses can detect and respond to anomalies instantly with AI, thus preventing potential damage before it escalates.
Improved Security & Fraud Detection
Threats of cybersecurity and financial fraud often come across as subtle anomalies in transactions or network activity. Ai powered detection assists in identifying such risks proactively.
Operational Efficiency & Cost Savings
When automating anomaly detection, businesses can lower manual efforts, create a seamless process and cut costs related to system failures or fraudulent activities.
Adaptability & Continuous Improvement
Not like static rule based detection, AI powered models improve overtime, adapting to new patterns and improving their detection.
The Roles of iPaaS in AI Anomaly Detection
Businesses who are looking to install AI powered anomaly detection at scale, iPaaS offers a simple and smooth way to integrate AI driven monitoring into your current system. Instead of wasting time on building complex integrations, businesses can rely on an iPaaS solution to centralize, optimize and automate their anomaly detection workflows.
Why Choose an iPaaS Over Native Integrations?
- Scalability – iPaaS solutions allow businesses to connect AI anomaly detection tools with multiple data sources, ensuring seamless scalability as data volumes grow.
- Flexibility – Unlike rigid native integrations, an iPaaS enables easy configuration and customization without the need for extensive coding.
- Speed – Businesses can deploy AI-powered anomaly detection faster through pre-built connectors, reducing the time needed for integration and implementation.
- Cost Efficiency – Maintaining native integrations can be expensive, requiring ongoing development and support. iPaaS eliminates these overhead costs.
Final Thoughts
AI powered anomaly detection is changing the way businesses safeguard their operations and optimize performance. Leveraging Ai and integrating it seamlessly with an iPaaS like Noca AI, businesses can grow their detection capabilities with ease. Enhance efficiency and gain a better insight into potential risks.